One of the key challenges in model – data fusion of dynamic systems is assess the most optimal data observations so that the performance of applied model – data fusion technique is maximized. This is crucially important especially in model – data fusion of distributed parameter systems, e.g. diffusion/convection process, due to possible restrictions on the number of applied data observation sensors or spatiotemporal variations of variable of interest. It is clear that poor data measurement, caused by misplacing the sensors over the domain of interest, results in poor estimate of variable of interest. Hence finding optimal location of the sensor is very important for an accurate model – data fusion.
Different techniques have been proposed to perform the task of data observation in presence of a spatiotemporally changing environment. Recently, multiple researches have been done on the field of optimal sensor placement, based on information theory.
The key idea is to optimally locate data monitoring sensors over the spatial domain of interest such that the uncertainty involved in parameter estimates is minimized. This has been achieved by maximizing the mutual information between the model output and data measurements.
where,
denotes the mutual information between model parameters and data observations. A dynamic programming based approach is used to maximize the mutual information between uncertain parameters and observational data. Proposed approach expedites the convergence of estimation process and avoids possible local optimalities while finding mobile sensor locations by maximizing the mutual information, rather moving along its gradient. In other words, proposed approach moves the mobile sensors toward the plume, even though the sensors are initially located far from the plume area. Along proposed approach for optimal sensor placement, a combination of generalized Polynomial Chaos (gPC) and Bayesian inference is used for data assimilation process which allows us to apply our method in presence of nonlinear dynamics and sensor model and non-Gaussian uncertainties, without using any Monte Carlo sampling.
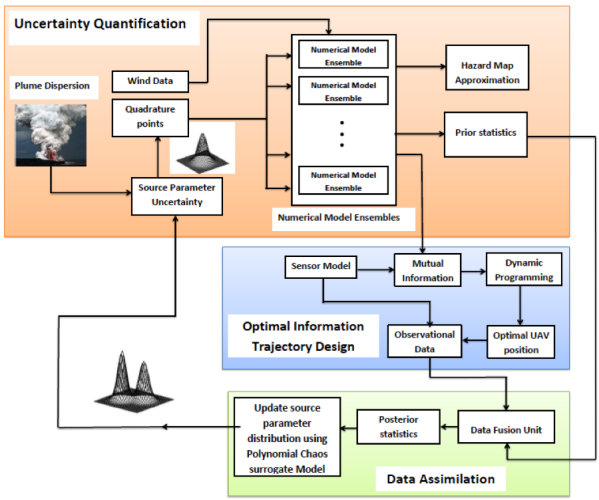
Schematic view of Optimal Information Collection estimation process and its connection with other components
A simple Example:
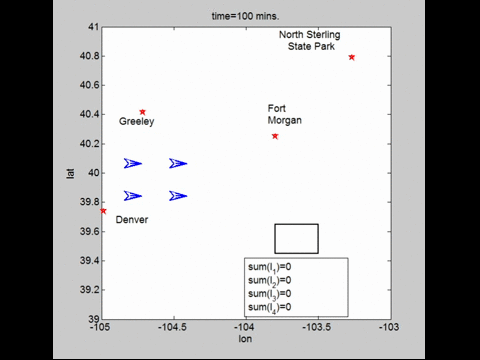
Smart Observations: Location of applied data observation sensors, determined based on maximizing the mutual information